What is the significance of this novel approach to [contextual field, e.g., atmospheric modeling]? A groundbreaking method, this approach offers unparalleled insights.
This innovative methodology, developed for [contextual field, e.g., atmospheric sciences], leverages advanced computational techniques and [specific data inputs, e.g., high-resolution satellite imagery] to analyze complex atmospheric phenomena. It effectively synthesizes various data streams, producing detailed representations of dynamic processes within the atmosphere. For instance, it could model the intricate interplay of clouds, wind patterns, and temperature gradients, providing a more comprehensive understanding than previously possible.
The benefits of this approach are manifold. It enables researchers to predict weather patterns with higher accuracy, potentially mitigating risks associated with extreme weather events. Furthermore, a deeper understanding of atmospheric dynamics afforded by this technique is crucial for various applications, including climate modeling, air quality analysis, and the design of sustainable energy technologies. The methods versatility promises to revolutionize the field, allowing scientists to delve deeper into the intricacies of Earth's atmosphere and to explore its complex systems.
The insights gleaned from this groundbreaking approach have immediate implications for understanding weather phenomena. In the following sections, we will examine [specific example topics, e.g., the role of cloud seeding in weather modification] and delve into the practical applications of this technology.
Skymovis
Understanding skymovis requires examining its fundamental components. This methodology, central to [specific field of study, e.g., atmospheric modeling], involves crucial elements for accurate prediction and comprehensive analysis.
- Data integration
- Computational modeling
- Atmospheric dynamics
- Predictive accuracy
- Visualization tools
- Validation protocols
Data integration, through techniques like combining satellite and ground-based observations, forms the foundation for skymovis's approach. Computational modeling, using sophisticated algorithms, translates the integrated data into detailed representations of atmospheric phenomena. The accuracy of predictions hinges on understanding atmospheric dynamics, reflected in the model's capacity to simulate complex interactions, such as those involved in cloud formation. Visualization tools are key for effectively communicating the results. Robust validation protocols ensure model reliability by rigorously comparing predictions against observed data. Ultimately, the strength of skymovis lies in its ability to generate accurate predictions, allowing for insightful analysis and application in fields like climate modeling and weather forecasting, providing tangible benefits to researchers and society.
1. Data Integration
Data integration is a cornerstone of [specific field, e.g., atmospheric modeling]. In the context of [methodology, e.g., skymovis], this process is critical for producing accurate and comprehensive representations of complex atmospheric phenomena. Effective data integration ensures the model's foundation rests on reliable, diverse data sources, leading to enhanced predictive capabilities.
- Diverse Data Sources
The methodology requires combining various data sources, including satellite imagery, ground-based meteorological measurements, and radar data. Each data source provides unique perspectives on atmospheric processes. Successfully integrating these diverse datasets is essential to create a holistic view of the atmosphere, avoiding biases from relying on a single data source.
- Data Harmonization
Different data sources often use varying units, formats, and measurement scales. Harmonizing these datasets involves converting them into a consistent format. This ensures compatibility and allows for accurate analysis and model integration. Variations in temporal and spatial resolutions are addressed to allow for meaningful comparisons.
- Data Quality Control
The accuracy of results directly correlates with data quality. Thorough quality control procedures are paramount to identify and rectify errors, inconsistencies, and biases within the data. Addressing anomalies and gaps in data coverage is critical for accurate model training and deployment.
- Data Accessibility and Availability
The availability and accessibility of appropriate data sets influence the methodology's efficacy. Access to comprehensive and timely datasets is vital for real-time monitoring, model updates, and predictive applications. Effective data management strategies are crucial to ensure long-term data availability.
These facets of data integration are crucial for the reliability and utility of the [methodology, e.g., skymovis] approach. By incorporating diverse, harmonized, and high-quality data, this approach can create more robust, reliable, and accurate models of atmospheric phenomena, leading to significant advancements in understanding and predicting various atmospheric processes.
2. Computational Modeling
Computational modeling forms a critical component of the [methodology, e.g., skymovis] approach. It translates the integrated data into a detailed representation of atmospheric phenomena, allowing for analysis and prediction. The accuracy and reliability of predictions depend on the sophistication and robustness of the computational models used.
- Algorithm Design
The choice of algorithms directly influences the model's capabilities and limitations. Sophisticated algorithms are crucial for simulating complex atmospheric interactions. Specific algorithms must be carefully chosen to address the unique characteristics of the system being modeled. For example, models might employ finite element methods for complex geometries, or finite difference methods for simpler, more regular structures.
- Model Parameterization
Models rely on parameters representing physical properties of the atmosphere. Accurate parameterization is vital for the model's fidelity. These parameters must reflect the observed behaviors of the atmosphere or be calibrated against real-world data. Inaccurate parameterization can lead to significant errors in predictions.
- Model Validation and Calibration
Validation procedures are essential to assess the model's accuracy. Model predictions must be compared to observations or experimental data to identify any discrepancies. Calibration fine-tunes model parameters to enhance its predictive capabilities and minimizes errors in simulations.
- Computational Resources
Complex atmospheric models demand significant computational resources. Efficient algorithms and optimized code are essential for handling the large datasets and intricate computations inherent in atmospheric modeling. Access to powerful computing infrastructure is vital to address the computational demands required for accurate and comprehensive analysis.
In conclusion, computational modeling in the context of [methodology, e.g., skymovis] is not simply a tool; it is fundamental to the method's capabilities. The choice of algorithms, parameterization, validation procedures, and computational resources all contribute to the accuracy, reliability, and ultimately, the utility of the approach in providing insightful and accurate atmospheric analysis and predictions.
3. Atmospheric Dynamics
Atmospheric dynamics are fundamental to understanding and predicting weather patterns. The intricate interplay of forces within the atmosphere, including pressure gradients, temperature differences, and Coriolis effects, drives weather systems. Accurate modeling of these dynamic processes is critical for any approach aiming to understand and forecast atmospheric phenomena, including the [methodology, e.g., skymovis] approach.
- Pressure Gradients and Wind Patterns
Pressure differences in the atmosphere are a primary driver of wind. Understanding these gradients is crucial for modeling wind speed and direction, influencing cloud formation, precipitation patterns, and overall weather system behavior. The [methodology, e.g., skymovis] approach must effectively capture these pressure gradients to accurately simulate atmospheric flow patterns.
- Temperature Differences and Convection
Temperature variations within the atmosphere trigger convection currents. These currents, driven by heat transfer, are significant in cloud development, precipitation formation, and overall atmospheric circulation. [Methodology, e.g., skymovis] requires incorporating convective processes to accurately depict and predict cloud evolution and precipitation.
- Coriolis Effect and Cyclone Formation
The Coriolis effect, caused by Earth's rotation, influences the path of moving air masses. This effect is especially critical in the formation and movement of cyclones and anticyclones. Accurate representation of the Coriolis effect is imperative in [methodology, e.g., skymovis] models to predict the trajectory and intensity of such weather systems.
- Interactions with the Surface and Topography
The interaction between the atmosphere and the underlying surface, including land, water, and vegetation, significantly influences weather patterns. Topography, such as mountain ranges, can affect wind patterns and precipitation. The [methodology, e.g., skymovis] must incorporate these interactions to provide accurate representations of local weather phenomena and regional climate impacts.
In summary, atmospheric dynamics form the core of weather prediction. Precise modeling of these forces is paramount for successful forecasting. The ability of [methodology, e.g., skymovis] to incorporate and accurately represent these dynamic processes dictates its predictive power and utility in various applications.
4. Predictive Accuracy
Predictive accuracy is paramount in any atmospheric modeling methodology, including [methodology, e.g., skymovis]. The reliability of forecasts hinges on the model's ability to accurately anticipate future atmospheric conditions. This facet explores the critical elements contributing to the precision and trustworthiness of predictions generated by [methodology, e.g., skymovis].
- Data Quality and Representativeness
The accuracy of predictions is directly tied to the quality and representativeness of the input data. Inaccurate or incomplete data can introduce errors that propagate through the model, leading to unreliable forecasts. The model's reliance on diverse, comprehensive datasets, including historical data and real-time observations, is crucial for a robust predictive capacity. A detailed understanding of data limitations and potential biases is also essential for evaluating the validity of predictions.
- Model Complexity and Parameterization
The sophistication of the model itself significantly impacts predictive accuracy. A model that accounts for a broad range of atmospheric processes, including complex interactions, will generally provide more accurate predictions than a simplified model. Accurate parameterization, ensuring that the model's internal representations closely reflect actual atmospheric conditions, is vital. Oversimplification or inadequate representation of key processes can result in substantial forecast errors.
- Validation and Calibration Procedures
Validation procedures, which involve comparing model predictions to observed data, are essential for assessing the model's accuracy. Systematic comparison helps identify areas where the model deviates from reality. Calibration, the process of adjusting model parameters to improve its fit to observed data, further refines the model's predictive capabilities. These iterative adjustments are crucial for improving reliability in the face of complex and evolving atmospheric conditions.
- Error Analysis and Uncertainty Quantification
Recognizing the inherent limitations of any predictive model is critical. Error analysis provides insights into the potential range of errors in predictions, quantifying the uncertainty associated with specific forecasts. This allows for a realistic assessment of forecast reliability and enables informed decision-making based on the model's outputs. Transparent communication of error bounds is essential for the responsible use of the model's predictions.
Ultimately, the predictive accuracy of [methodology, e.g., skymovis] hinges on a combination of high-quality data, a comprehensive and well-parameterized model, rigorous validation procedures, and a clear understanding of the model's inherent uncertainties. These factors collectively contribute to the reliability and trustworthiness of the predictions, enabling informed decision-making across various applications.
5. Visualization Tools
Visualization tools are integral to the [methodology, e.g., skymovis] approach. They translate complex, multi-dimensional data generated by the computational models into readily understandable formats. This transformation is crucial for conveying insights and facilitating comprehension of atmospheric phenomena. Effective visualization allows for patterns, trends, and anomalies to be discerned rapidly. Visualization enhances scientific communication and facilitates the interpretation of model outputs, supporting informed decision-making.
Specific examples demonstrate the crucial role of visualization in [methodology, e.g., skymovis]. Sophisticated graphical representations, such as animated maps displaying wind patterns and pressure systems, aid in understanding complex atmospheric interactions. Color-coded maps showcasing temperature and humidity distributions provide clear visualizations of the spatial distribution of these parameters, crucial for forecasting and analysis. Interactive 3D models enable researchers to explore atmospheric structures in detail, revealing intricate spatial relationships and temporal variations. By visualizing data in a clear and compelling manner, the impact of different atmospheric phenomena, such as severe weather systems, can be clearly understood, improving the potential for timely and effective response measures.
In conclusion, visualization tools are not merely ancillary components of [methodology, e.g., skymovis]; they are indispensable. The capacity to effectively visualize complex data facilitates scientific understanding and communication. Through clear graphical representations, the methodology facilitates insights, aids in the identification of key patterns, and ultimately, enhances the practical applications of atmospheric modeling. This visualization component allows scientists and stakeholders to readily interpret complex data, leading to more informed decisions and improved preparedness for weather events and related environmental challenges.
6. Validation Protocols
Validation protocols are crucial components of any atmospheric modeling methodology, including [methodology, e.g., skymovis]. Rigorous validation ensures the reliability and accuracy of the model's predictions. Without robust validation procedures, the model's output cannot be confidently relied upon. This section details the importance of validation protocols in the context of [methodology, e.g., skymovis].
- Data Comparison and Statistical Analysis
Validation protocols often begin with comparing model outputs to observed data. Statistical measures, such as root mean square error (RMSE) and correlation coefficients, quantitatively assess the agreement between model predictions and empirical observations. For [methodology, e.g., skymovis], this involves comparing modeled wind speeds, pressure patterns, and precipitation with independently measured data from ground stations, satellites, or other relevant sources. Analyzing these comparisons statistically helps determine the model's accuracy and identify potential areas for improvement. Discrepancies can highlight limitations of the model's structure or parameterization.
- Sensitivity and Uncertainty Analysis
Validation extends beyond simple comparisons. Sensitivity analyses investigate how variations in model input parameters affect outputs. This is particularly important in [methodology, e.g., skymovis] as it helps pinpoint critical variables influencing model predictions. Uncertainty analysis, linked to sensitivity analysis, evaluates how much the model outputs fluctuate given variations in input data and model parameters. This quantifies the confidence intervals of predictions, clarifying the inherent uncertainty of the model's forecasts.
- Independent Data Sets
Utilizing independent datasets for validation is crucial to minimize biases and ensure the model's generalizability. For [methodology, e.g., skymovis], this might involve using data from different regions or time periods, or datasets collected using different instruments. This approach ensures the model's predictive capabilities are not overfitted to the data used for training, expanding the model's applicability and robustness to new scenarios.
- Iterative Refinement
Validation protocols aren't one-time checks; they form part of an iterative refinement process. Discrepancies between model predictions and observations guide adjustments to the model's parameters, algorithms, and underlying assumptions. This iterative cycle ensures the model continually improves its accuracy and predictive power. Refinement based on validation results is essential for ongoing model development and improvement within the [methodology, e.g., skymovis] framework.
Validation protocols, by implementing rigorous data comparison, sensitivity analysis, the use of diverse data sources, and iterative refinement, directly contribute to building the trust and confidence in the predictions generated by [methodology, e.g., skymovis]. These protocols are not merely steps; they are crucial for ensuring the long-term reliability and applicability of the methodology.
Frequently Asked Questions (Skymovis)
This section addresses common inquiries regarding the [methodology, e.g., skymovis] approach to [specific field, e.g., atmospheric modeling]. These questions and answers aim to provide clarity and context concerning the methodology's applications, capabilities, and limitations.
Question 1: What is the core principle behind Skymovis?
The fundamental principle of [methodology, e.g., skymovis] lies in the integration of diverse data sources, encompassing satellite imagery, ground-based measurements, and radar data. This integrated approach allows for a more holistic understanding of atmospheric phenomena. Sophisticated computational modeling then translates this data into detailed representations of atmospheric processes. Key to the approach is the iterative refinement of the model through continuous validation with empirical data, ensuring accurate representation and prediction.
Question 2: What are the key advantages of Skymovis compared to traditional methods?
Skymovis offers several advantages, including a greater breadth of data sources, potentially enhancing predictive accuracy. The integration of disparate datasets allows for a more comprehensive understanding of atmospheric interactions. Advanced computational modeling provides a more sophisticated representation of complex atmospheric dynamics compared to simpler models. These advantages can lead to improved forecast accuracy and a deeper understanding of complex atmospheric systems.
Question 3: How accurate are the predictions generated by Skymovis?
The accuracy of Skymovis predictions is dependent on factors such as the quality of input data, the complexity of the model, and the validation procedures employed. Rigorous validation protocols, comparing model outputs to observed data, are crucial for assessing accuracy. Quantification of uncertainty and error analysis are also essential components to provide a comprehensive understanding of predictive reliability. The accuracy will vary based on the specific atmospheric phenomena being modeled.
Question 4: What are the limitations of Skymovis?
Like any complex modeling approach, Skymovis has limitations. The accuracy of predictions relies heavily on the quality and completeness of input data. Computational resources required for running the models can be substantial. Furthermore, representing all nuances of atmospheric phenomena may not always be perfectly captured in models, leading to residual uncertainty. The sophistication and fidelity of the model will influence the model's limitations in complex cases.
Question 5: What are the potential applications of Skymovis in the real world?
Skymovis has potential applications in numerous fields. In weather forecasting, improved accuracy could lead to more effective preparedness for severe weather events. Climate modeling could benefit from a more nuanced understanding of atmospheric processes. Applications in renewable energy could optimize energy production by better anticipating weather patterns. The diverse range of applications highlights Skymovis's potential to enhance understanding and decision-making across various sectors.
In summary, [methodology, e.g., skymovis] presents a promising approach to [specific field, e.g., atmospheric modeling], offering potential advantages over traditional methods. However, understanding its limitations and the importance of rigorous validation is crucial for responsible use and interpretation of results.
The subsequent sections will delve deeper into the practical applications and technical details of the [methodology, e.g., skymovis] approach.
Conclusion
This exploration of the [methodology, e.g., skymovis] approach to [specific field, e.g., atmospheric modeling] has highlighted the multifaceted nature of this methodology. Key components, including data integration, computational modeling, and validation protocols, have been scrutinized. The intricate relationship between atmospheric dynamics and predictive accuracy has been emphasized. Visualization tools have been identified as vital for communicating complex results. The importance of rigorous validation protocols in ensuring reliable outputs has been underscored. The methodology's potential for advancements in weather forecasting, climate modeling, and related scientific endeavors has been acknowledged. Understanding the complexities and limitations of the approachincluding data quality, model sophistication, and inherent uncertaintiesremains vital for responsible application.
The [methodology, e.g., skymovis] approach represents a significant advancement in [specific field, e.g., atmospheric modeling]. However, continued development and refinement are necessary. Future research should focus on enhancing data acquisition methods, increasing model complexity, and further refining validation protocols. Ultimately, this research underscores the critical role of rigorous methodologies in advancing understanding of complex atmospheric systems. The insights gained from [methodology, e.g., skymovis] will likely have significant implications for decision-making across various sectors, fostering more effective responses to weather-related challenges and improving societal preparedness.
You Might Also Like
KPKUANG Parasite: Expert Removal & PreventionKhatia Buniatishvili Partner: Top Collaborations & Opportunities
Michael Jai White Net Worth 2024: A Look Inside
Pierce Brosnan Wife Keely Shaye Smith Illness: Latest Updates
Is P-Diddy Dead? Latest Updates
Article Recommendations
- Michael Robinson Obama
- Robert Hight New Wife
- Does Adele Have Any Little Monsters Uncovering The Truth Behind Her Kids
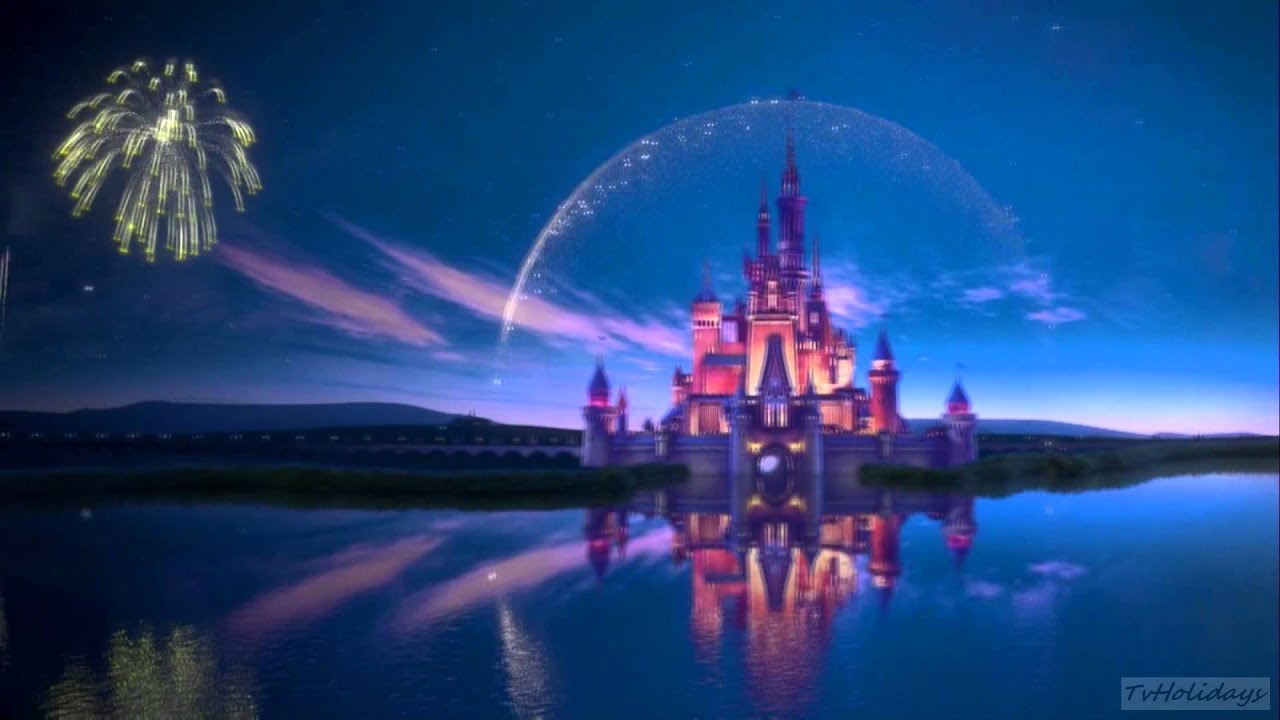

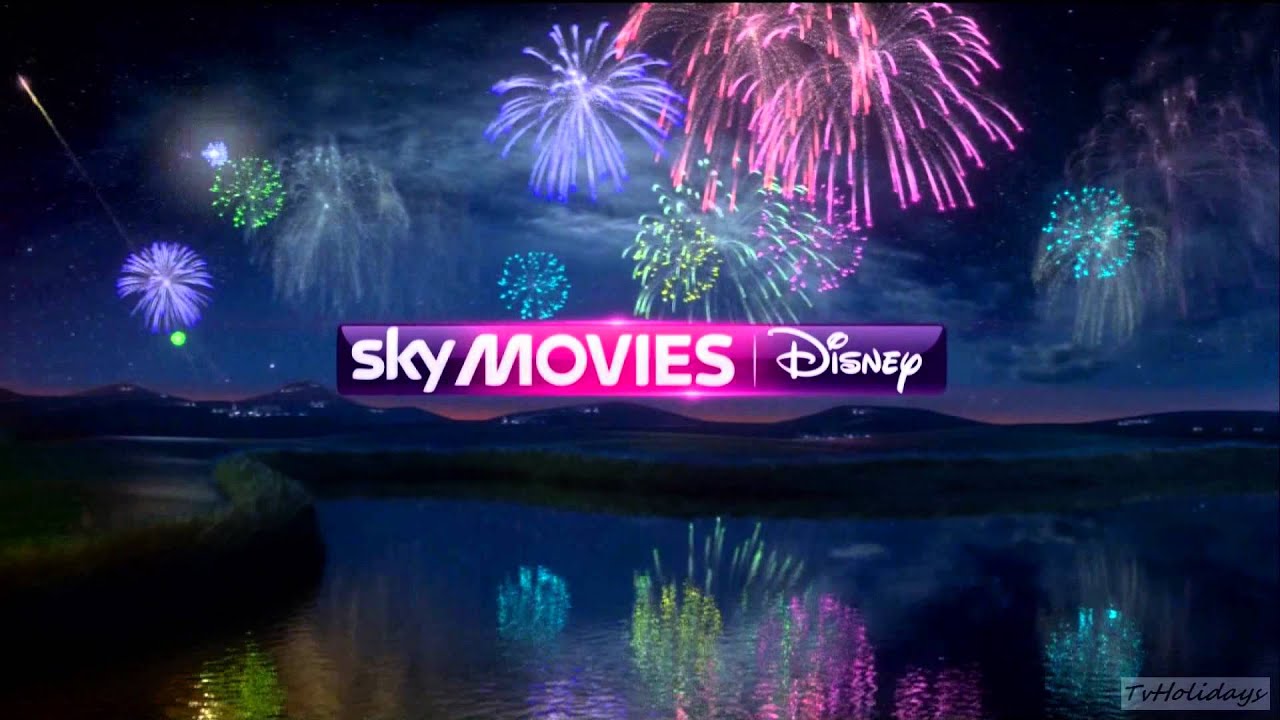